In 2022, I paused my blog to accept a job offer, where I developed new products. In 2023, I attempted to resume writing but found balancing the role and blogging challenging. Only after dedicating time to creating a solution with AI's support was I able to return to blogging in 2024. AI has revolutionized the tech industry, as has past internet and mobile technology innovations. Early tech investments, such as those of Microsoft and Apple, have shown how transformative such breakthroughs can be.
Resuming my blog wasn't easy. Writing consistently while managing my hedge fund responsibilities was daunting. I invested heavily in APIs of Large Language Models (LLM) to build Python frameworks, transforming my blogging process. A backend now generates all financial information on the blog I developed over the past 12 months. This automation has streamlined updates, allowing me to seamlessly study and write on diverse topics. Previously, I spent about 70% of my time on Excel and 30% on writing. I spend around 80% of my time on content and writing while the backend handles data processing. Although AI isn't perfect for every task, it has been an excellent teacher, enhancing my Python skills and enabling the creation of a robust framework in a few months.
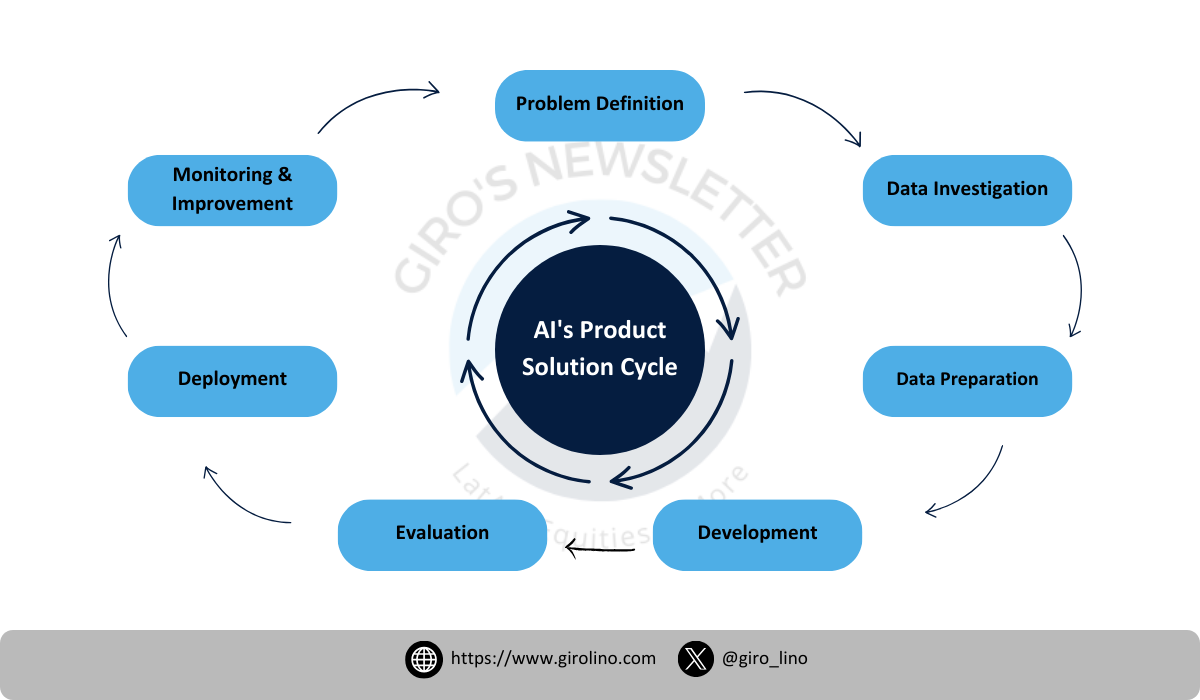
Although AI isn't perfect for every task, it has been an excellent teacher. It has enhanced my Python skills and enabled the creation of a robust framework in a few months. AI, encompassing machine learning, neural networks, and deep learning, has revolutionized my workflow. The diagram below shows the broad concept of AI and its components.
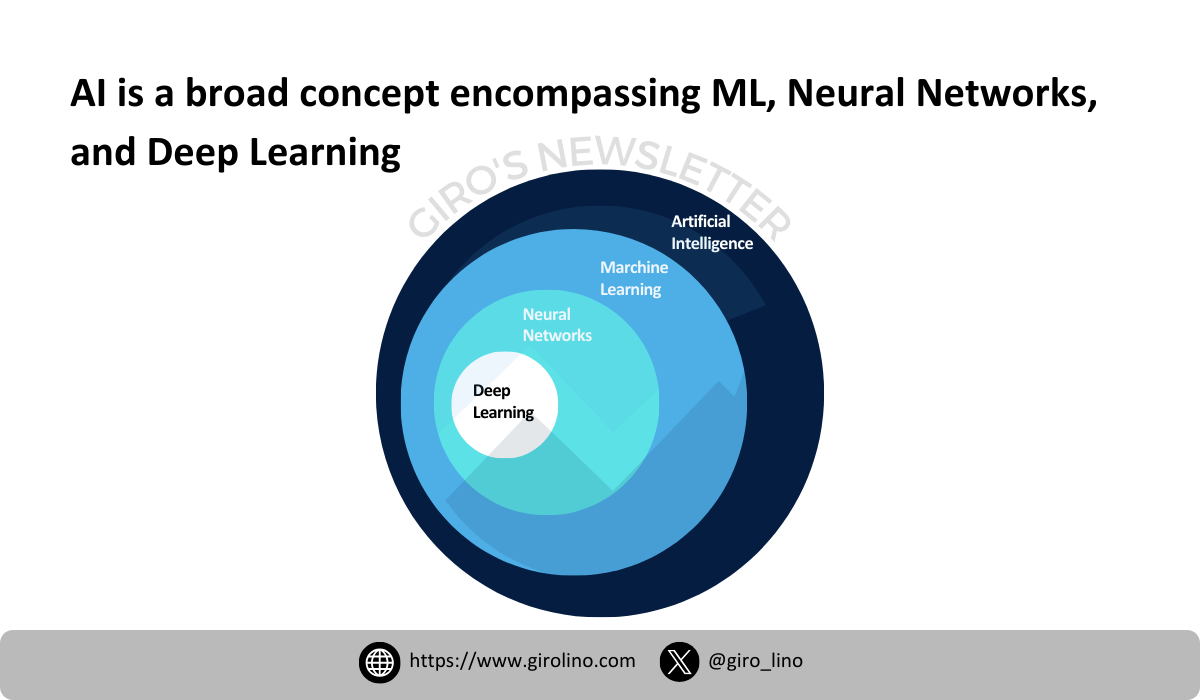
The potential of AI in investment strategies is immense. For individual investors and institutions like hedge funds, AI can provide deeper insights, automate tedious tasks, and facilitate better decision-making processes. The journey of learning and leveraging AI underscores its transformative power and highlights the importance of continuous adaptation in the ever-evolving investment landscape.
My journey from a solo blogger to working at a hedge fund and back to blogging with AI's support showcases the dynamic nature of AI investments. AI has made this dual role possible, emphasizing its role as a powerful enabler. For readers, considering AI in their investment strategies could be a game-changer. Continuous learning and embracing AI's potential are crucial for staying ahead in the investment world.
Understanding the IPA Computing Cycle
In recent years, Artificial Intelligence (AI) has experienced unprecedented growth and attention, capturing the imagination of technologists, businesses, and the general public. To fully grasp AI's current state and future potential, we'll examine it through the lens of the IPA computing cycle - a framework for Infrastructure, Platforms, and Applications.
The IPA cycle posits that major technological shifts unfold in three distinct, often overlapping phases:
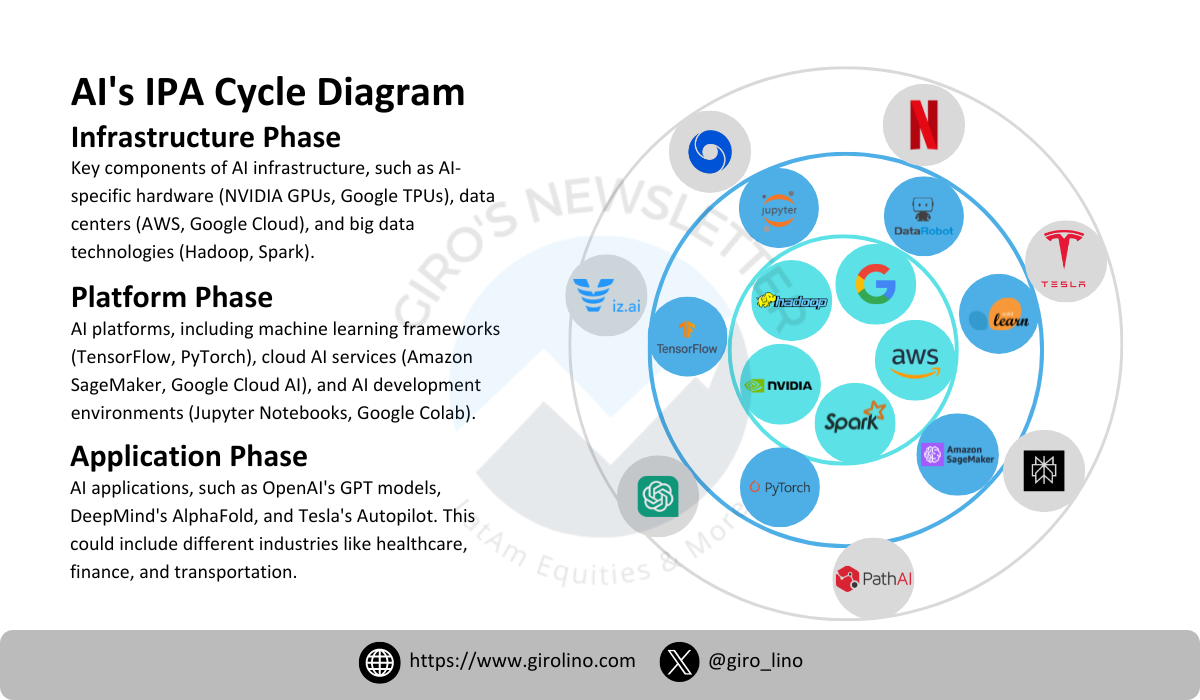
These phases aren't strictly sequential; they interact and overlap, with advancements in one area often driving improvements in others. As one cycle matures, it sets the stage for the next technological revolution, creating a continuous innovation process.
By understanding where AI stands in this cycle, we can better anticipate its future developments, challenges, and opportunities. In this post, we'll break down each phase of the IPA cycle, examine historical examples, and analyze AI's current position within this framework. We'll also discuss AI's unique challenges and opportunities and speculate on its future trajectory.
Whether you're a tech enthusiast, a business leader, or simply curious about AI's future, understanding the IPA cycle will provide valuable context for the AI revolution unfolding before us. Join us as we embark on this journey through AI's past, present, and future, viewed through the illuminating lens of the IPA computing cycle.
Historical Examples of IPA Cycles
Examining how this pattern has played out in previous technological revolutions is helpful to better understand how the IPA cycle applies to AI. Let's look at three significant examples: the Personal Computer Revolution, the Internet Age, and the Mobile Era.
The Personal Computer Revolution
Infrastructure: The PC revolution began in the 1970s with the development of microprocessors and memory chips. Companies like Intel and Motorola created increasingly powerful and affordable CPUs, while others focused on storage technologies.
Platforms: Operating systems like MS-DOS and early Windows and Mac OS versions emerged as platforms. These provided a standardized environment for software development and user interaction.
Applications: Platform availability led to the explosion of software applications in the 1980s and 1990s. Word processors, spreadsheets, and games became commonplace, driving the widespread adoption of personal computers.
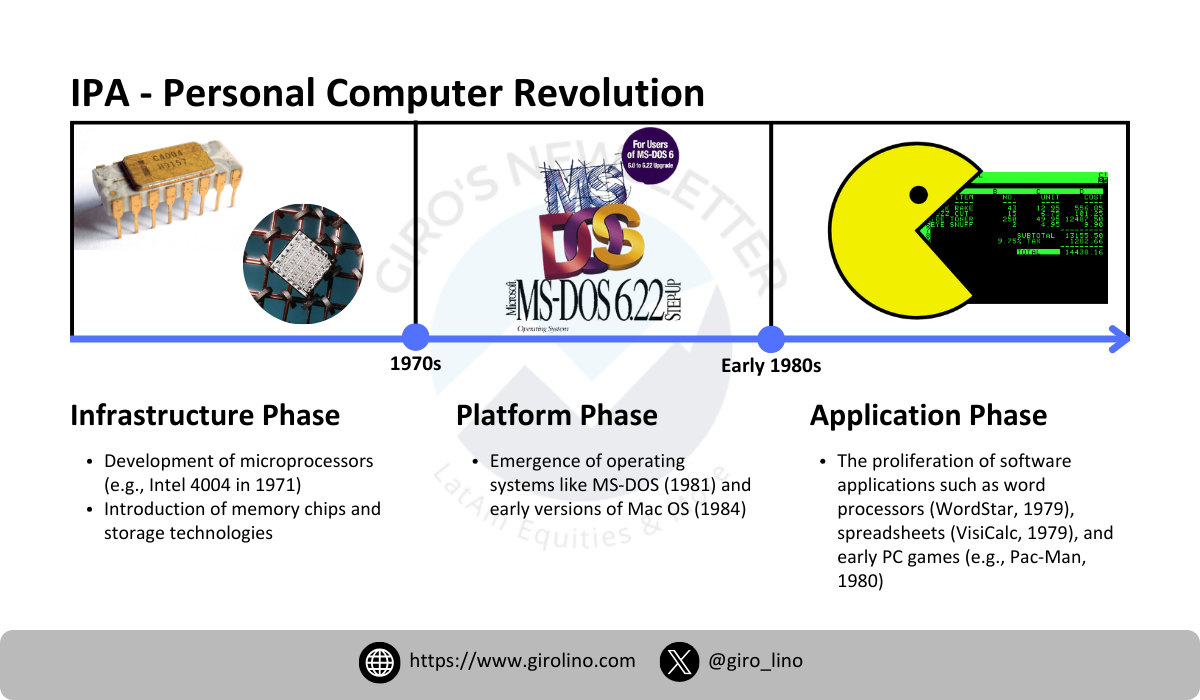
The Internet Age
Infrastructure: The Internet's infrastructure phase involved the development of networking protocols (TCP/IP), creating the World Wide Web, and rolling out broadband connections.
Platforms: Web browsers like Netscape Navigator and Internet Explorer became crucial platforms, along with web development technologies like HTML, CSS, and JavaScript. Later, more sophisticated platforms like PHP, Java, and .NET frameworks emerged.
Applications: The application phase saw the rise of e-commerce (Amazon), search engines (Google), social media (Facebook), and countless other web-based services that transformed how we interact, work, and do business.
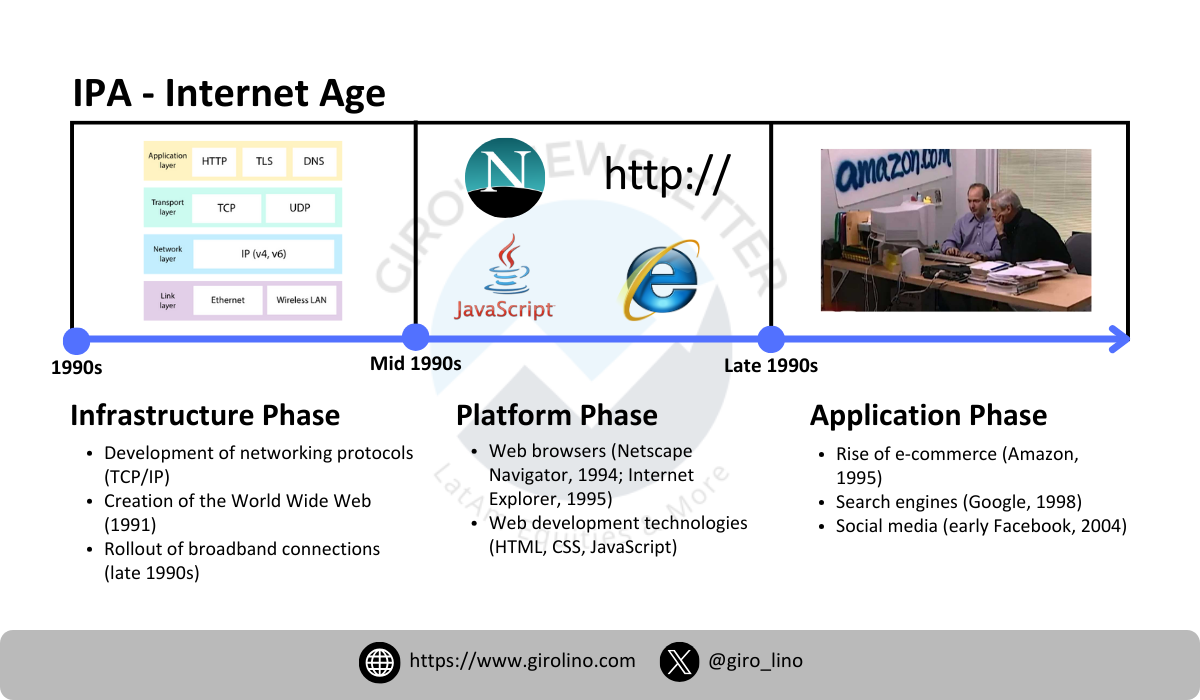
The Mobile Era
Infrastructure: The mobile revolution was built on advancements in smartphone hardware, including touchscreens, mobile processors, and cellular networks (3G, 4G, and now 5G).
Platforms: Mobile operating systems, particularly iOS and Android, became the dominant platforms. These provided SDKs (Software Development Kits) and app stores, making it easy for developers to create and distribute mobile applications.
Applications: The app ecosystem exploded, with millions covering every conceivable need, from communication and productivity to entertainment and health tracking. This led to the mobile-first approach we see in many industries today.
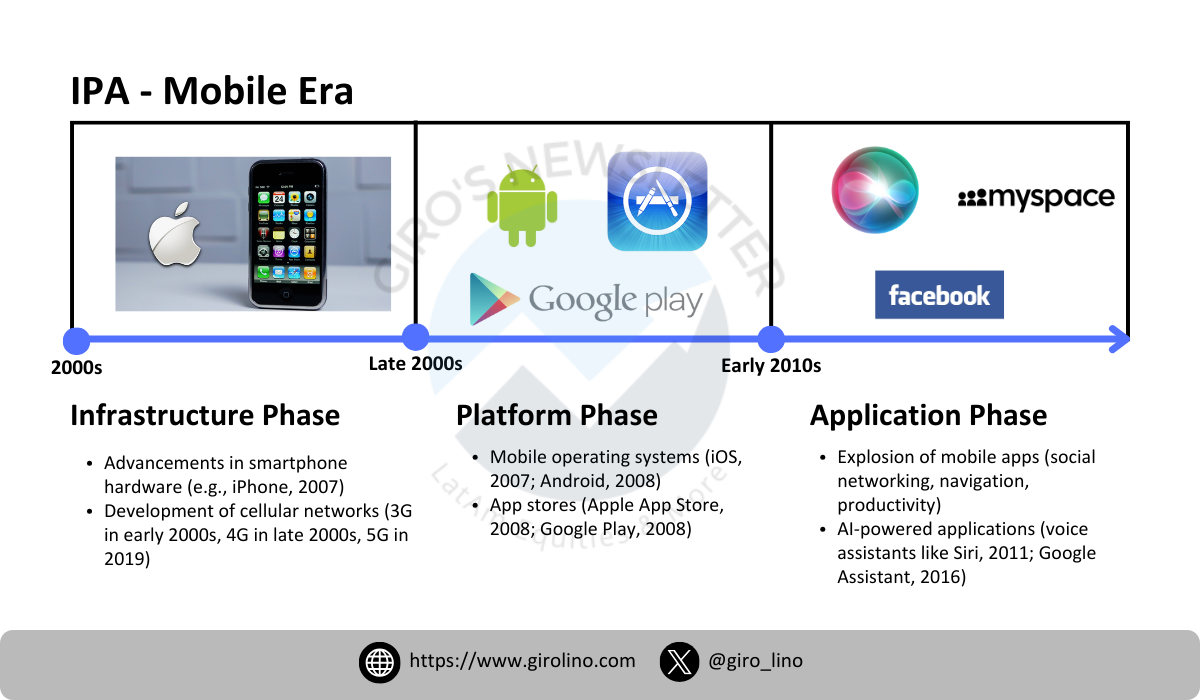
Current AI Progress Through the IPA Lens
We can see the IPA cycle in action by examining the current state of AI development. Let's break down each phase to understand where AI stands today:
Infrastructure: The Foundation of AI
The infrastructure phase of AI is well underway and rapidly evolving. Key components include:
- AI Chips: Companies like NVIDIA, AMD, and Google are developing specialized processors (GPUs, TPUs) optimized for AI workloads. These chips are crucial for handling the massive computational requirements of AI models.
- Data Centers: Cloud providers such as Amazon Web Services, Microsoft Azure, and Google Cloud are expanding their data center capacities to meet the growing demand for AI computing power.
- Big Data Technologies: Infrastructure for collecting, storing, and processing vast data is essential for training AI models. Technologies like Hadoop, Spark, and NoSQL databases form the backbone of this data infrastructure.
- High-Speed Networks: The rollout of 5G networks and advancements in fiber optic technology enable faster data transmission, critical for real-time AI applications.
Emerging Platforms: Building the AI Ecosystem
While still evolving, several key platforms are emerging in the AI space:
- Machine Learning Frameworks: TensorFlow, PyTorch, and sci-kit-learn have become standard tools for developing AI models, providing high-level APIs that abstract away much of the underlying complexity.
- Cloud AI Services: Major cloud providers offer AI-as-a-service platforms, allowing developers to access pre-trained models and AI capabilities without building everything from scratch.
- AI Development Environments: Integrated development environments (IDEs) specifically designed for AI, such as Jupyter Notebooks and Google Colab, are streamlining the AI development process.
- AI Model Marketplaces: Platforms like Hugging Face and NVIDIA NGC are emerging as repositories for pre-trained AI models, enabling faster development and deployment of AI applications.
Early Applications: The First Wave of AI Products
While we're still in the early stages of the application phase, several groundbreaking AI applications have already emerged:
- Natural Language Processing: Applications like ChatGPT and GPT-4 have demonstrated impressive language understanding and generation capabilities.
- Computer Vision: AI-powered image and video analysis is used in various fields, from autonomous vehicles to medical imaging.
- Recommendation Systems: AI algorithms power personalized recommendations on platforms like Netflix, Spotify, and Amazon.
- AI-assisted Creativity: Tools like DALL-E and Midjourney push the boundaries of AI-generated art and design.
- Autonomous Systems: From self-driving cars to robotic process automation, AI enables new levels of autonomy in various domains.
The rapid adoption of AI applications is particularly noteworthy. ChatGPT, developed by OpenAI, exemplifies this unprecedented growth. As illustrated in the following image, ChatGPT reached 100 million users in just three months, making it the fastest-growing consumer application in history.
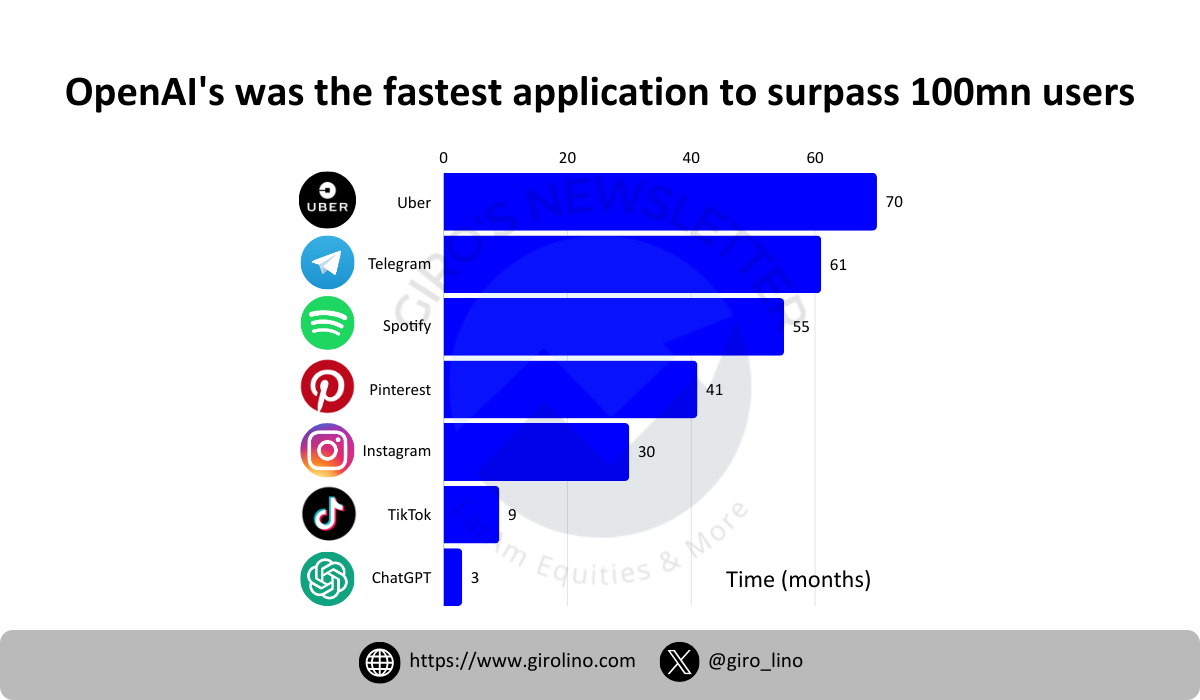
This chart highlights the extraordinary pace of AI adoption and demonstrates how AI applications can gain traction even as the underlying infrastructure continues to evolve. It's a testament to AI's transformative potential and the public's eagerness to engage with this technology.
The swift rise of ChatGPT and similar AI applications underscores the importance of the infrastructure and platform phases of the IPA cycle. Without the foundational work in these earlier phases, such rapid deployment and adoption of AI applications would not be possible. The recent introduction of ChatGPT-4o, an advanced iteration of the GPT model, further exemplifies this trend. ChatGPT-4o's enhanced capabilities in natural language processing, multi-modal interactions, and complex problem-solving demonstrate the rapid pace of AI evolution.
Its improved performance in financial analysis and investment strategy formulation highlights the growing potential of AI in the investment sector. At the same time, the success of these applications drives further investment and development in AI infrastructure and platforms, creating a positive feedback loop that accelerates progress across all phases of the IPA cycle. The widespread adoption and application of tools like ChatGPT-4o in various industries, including finance and investment, further fuels this cycle of innovation and improvement.
AI development is challenging our expectations of the traditional IPA cycle. Here are some surprising facts:
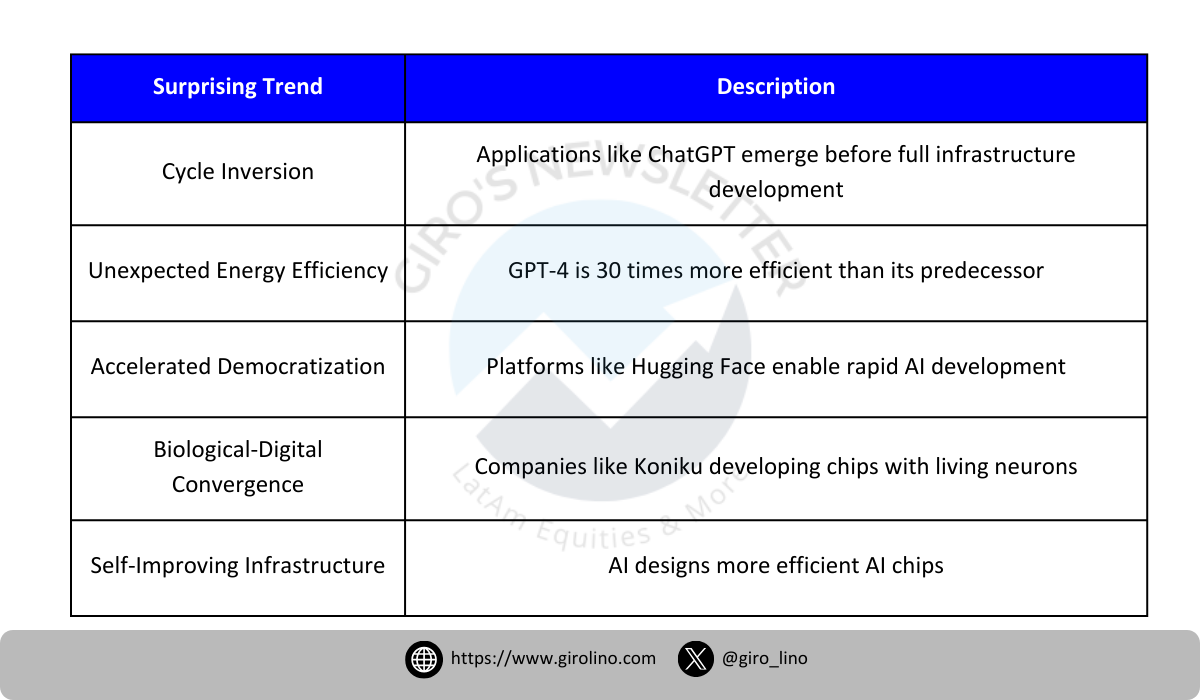
These trends indicate that the AI IPA cycle is developing more complex and interconnectedly than any previous technological revolution, promising even faster and more unpredictable changes in the near future.
Case Study: AI Infrastructure Investment
The massive investments in AI infrastructure highlighted in this case study have significant implications for AI stocks. Companies at the forefront of AI chip development, cloud computing, and AI services are likely to benefit from this trend. For instance:
- Chip Manufacturers: Companies like NVIDIA, AMD, and Intel, which are developing specialized AI chips, could see substantial growth. NVIDIA, in particular, has become a bellwether AI stock due to its dominance in GPU technology crucial for AI computing.
- Cloud Service Providers: Major cloud players like Microsoft, Amazon (AWS), and Google (Alphabet) are poised to benefit from the increased demand for AI computing resources. Their stock performance may be influenced by their ability to scale AI infrastructure effectively.
- AI-Focused Startups: Emerging companies specializing in AI hardware or software solutions could become attractive investment targets. As they go public, they may offer high-growth potential in the AI stock market.
- Traditional Tech Companies: Established tech firms investing heavily in AI, like IBM or Salesforce, might see their stock valuations affected by their success in integrating AI into their product offerings.
- ETFs and Index Funds: For investors looking for broader exposure, AI-focused ETFs that include a basket of companies involved in AI infrastructure and development could be an option.
It's important to note that while the growth potential for AI stocks is significant, the market is also highly competitive and can be volatile. Investors should carefully consider factors such as a company's financial health, market position, and long-term AI strategy when evaluating AI stocks.
Moreover, the success of AI infrastructure investments could create a ripple effect, potentially boosting stocks in sectors that benefit from advanced AI capabilities, such as healthcare, finance, and autonomous vehicles.
To illustrate the scale of investment in AI infrastructure, let's examine a hypothetical model based on industry trends, which could represent Microsoft's approach to AI investment. This model projects ambitious growth in AI-related services, with revenues potentially surging from $1.7 billion in the first year to over $111 billion by the tenth year, representing a compound annual growth rate of about 59%.
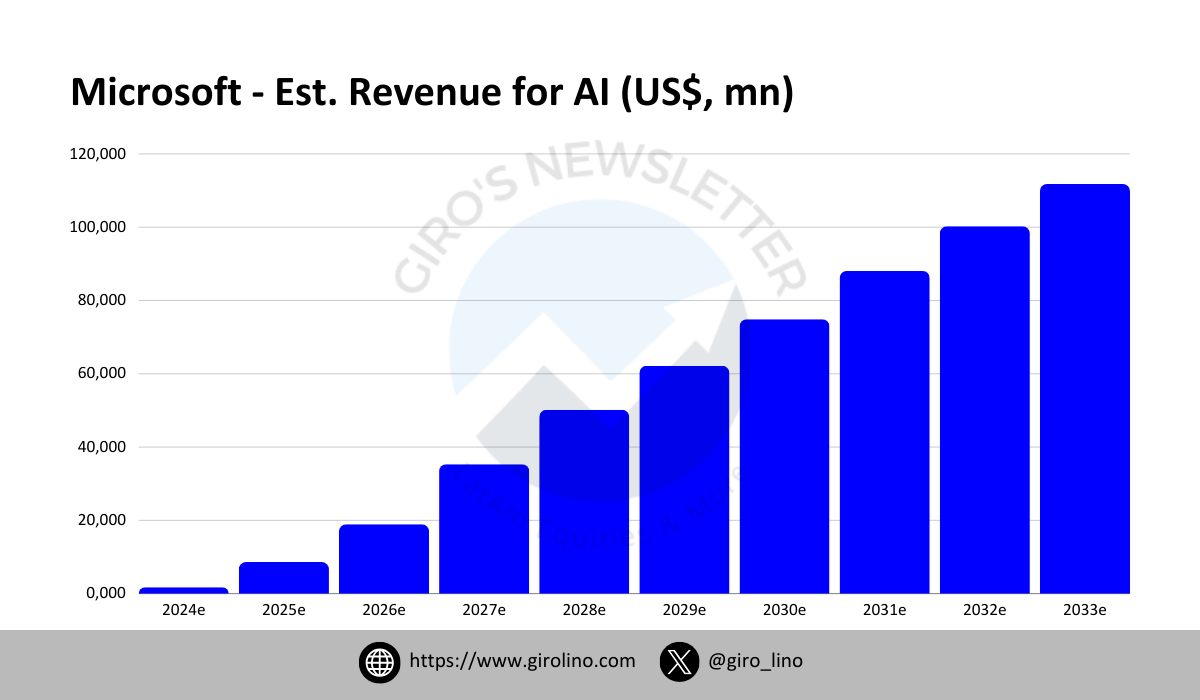
To support this extraordinary growth, the company plans to expand its AI computing capacity dramatically. The model envisions an increase from 3.42 million units in the first year to over 271 million units by year ten, with particularly aggressive growth in the early years. This massive scale-up requires substantial capital expenditure, peaking at over $42 billion in the fifth year before gradually decreasing. Over the decade, the cumulative capital expenditure is projected to reach $280 billion.
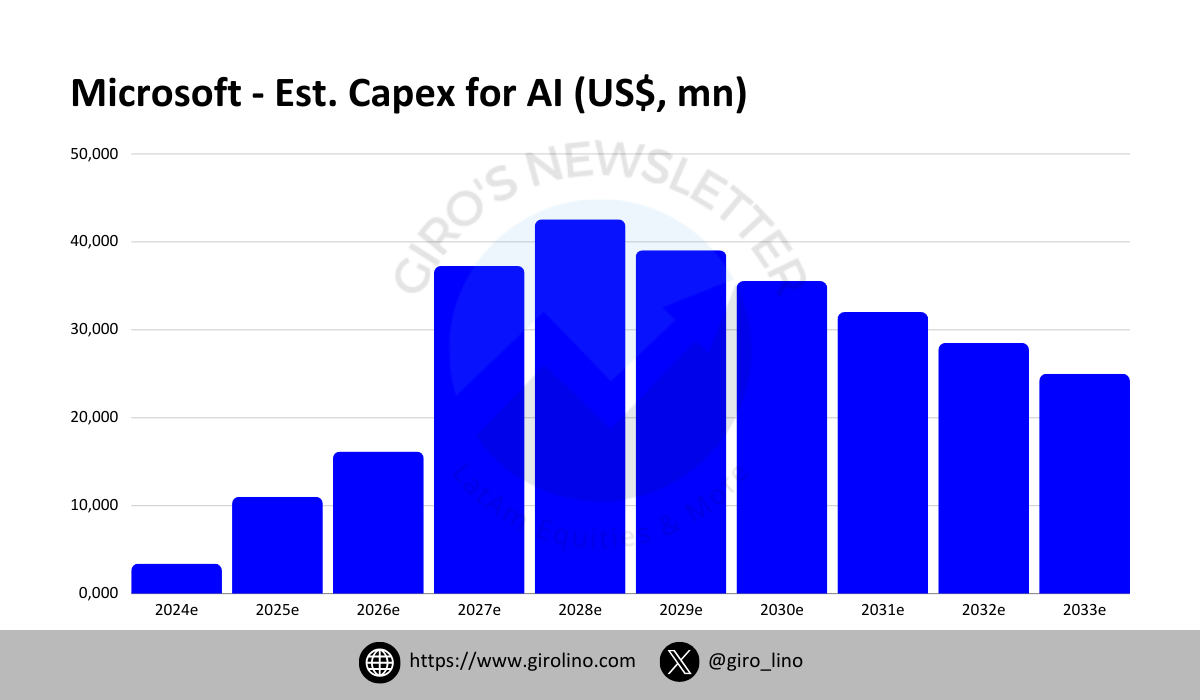
The investment spans various critical infrastructure areas. The largest portion is allocated to servers and computing hardware, reaching $17 billion at its peak. Significant funds are also directed toward data center buildings, specialized AI hardware, and power and cooling systems. This comprehensive approach underscores the complexity of building AI infrastructure, requiring investments in AI-specific components and the entire ecosystem necessary for advanced AI capabilities.
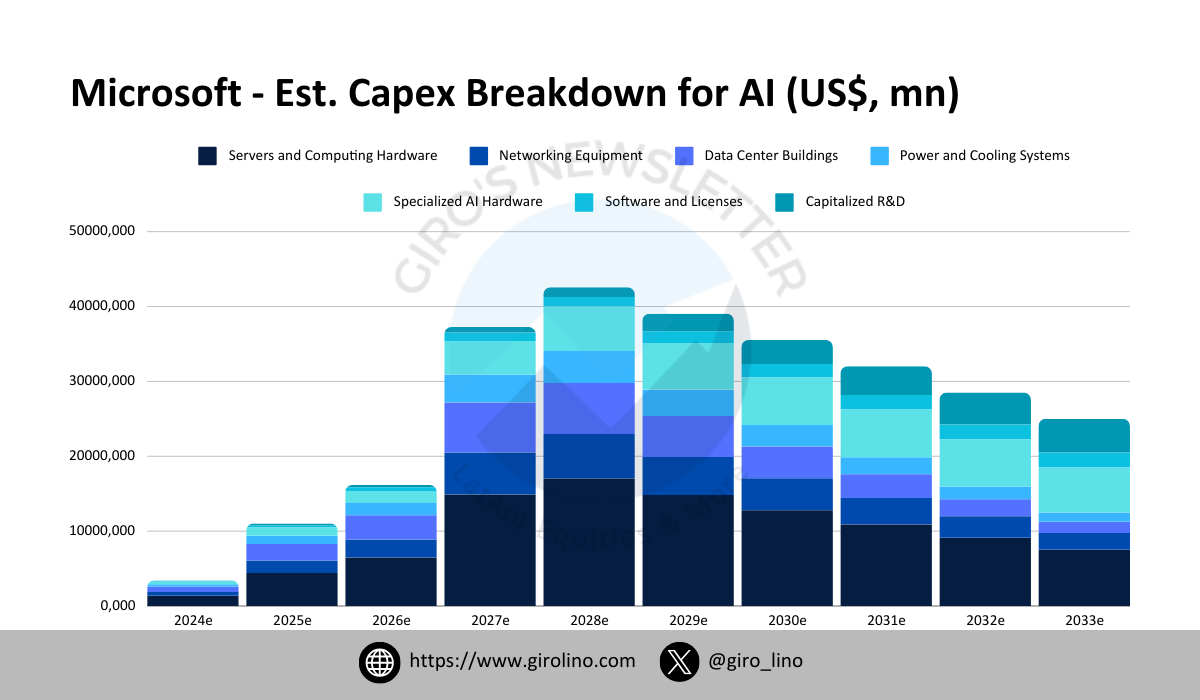
Despite these enormous upfront costs, the model projects a path to profitability. The company is expected to become profitable by the fourth year, with margins expanding significantly afterward. The financial trajectory suggests negative free cash flow for the first five years due to heavy investments, turning positive in the sixth year and reaching over $40 billion by year ten.
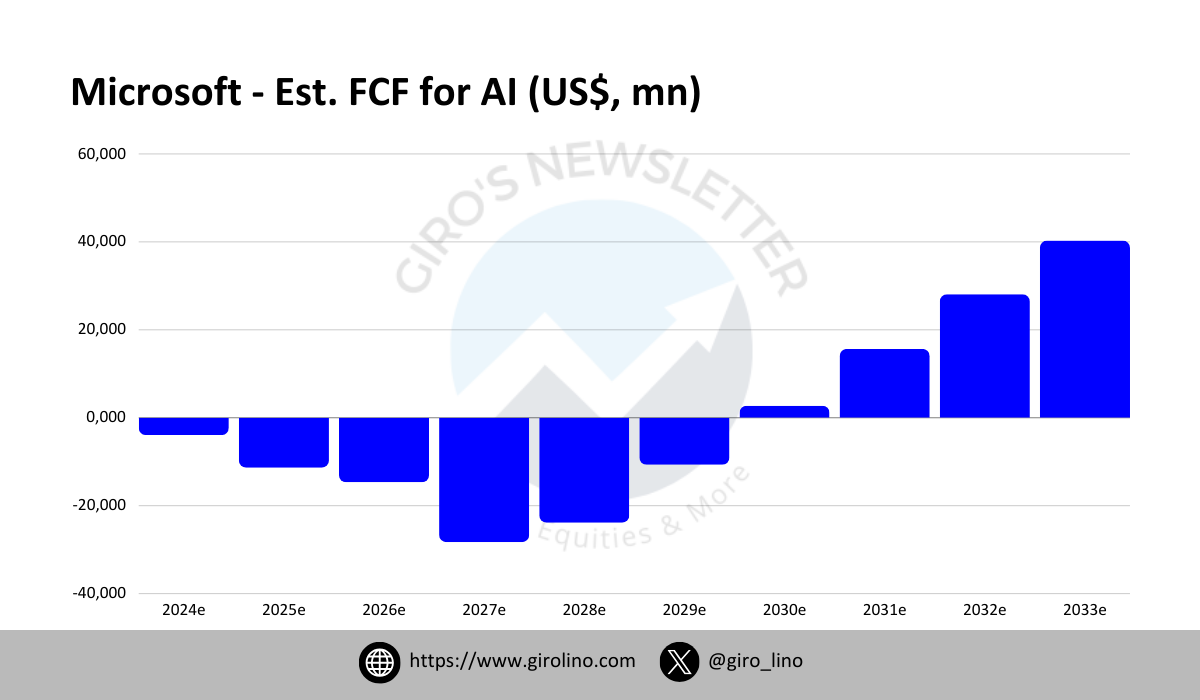
This hypothetical case study highlights our estimate for Microsoft's long-term perspective toward AI. They believe these substantial initial investments will yield significant returns through new revenue streams and increased efficiency. The projected financial path aligns with the IPA cycle we've discussed, where early infrastructure investments lay the groundwork for subsequent platform development and ultimately lead to a proliferation of applications and services.
The scale of investment and the potential returns illustrated in this model provide a concrete context for our discussion of AI's current progress, particularly in the infrastructure phase. It also sets the stage for our upcoming exploration of the challenges and opportunities in AI development and our predictions for the future of this transformative technology.
Comparing AI to Previous Cycles
AI's progression through the IPA cycle shares similarities with past technological revolutions but also presents unique characteristics. This comparison provides insights into AI's potential trajectory and development:
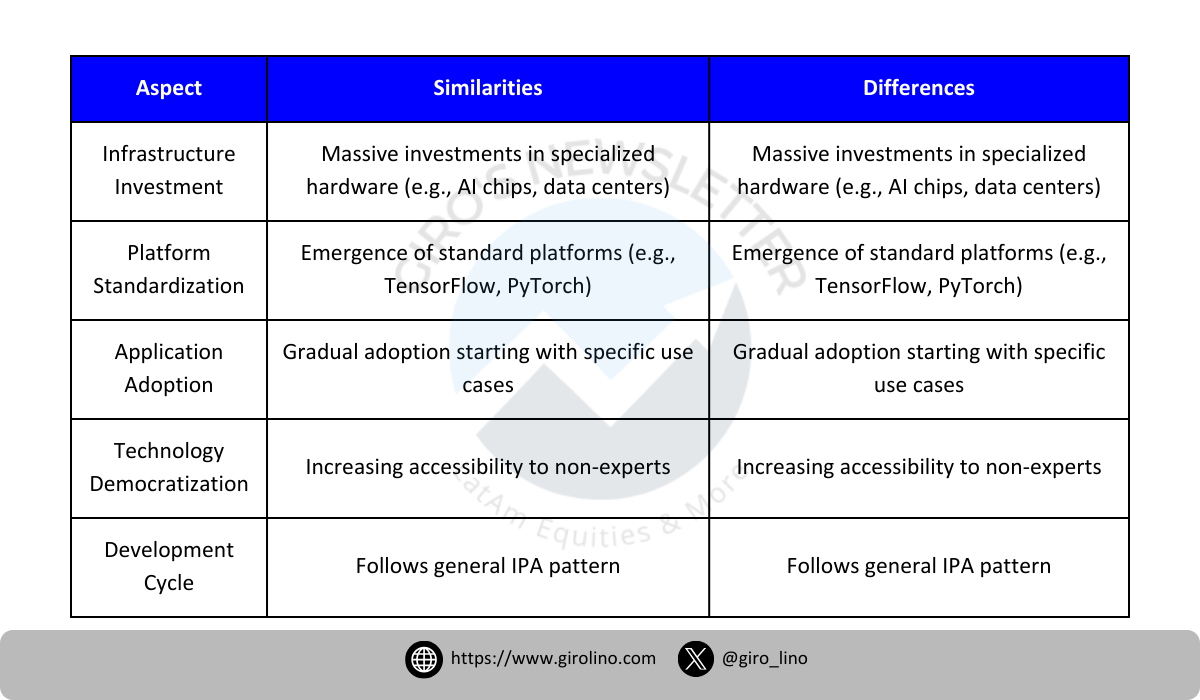
Based on previous cycles and the current state of AI, we can make some educated guesses about its future timeline:
- Infrastructure Phase (2015-2025): We're currently in the latter stages of this phase, with continued investments in AI chips, cloud computing, and data Infrastructure.
- Platform Phase (2020-2030): This phase is ramping up, with AI development platforms becoming more sophisticated and accessible. We expect this phase to mature over the next 5-10 years.
- Application Phase (2025-2040+): While some AI applications are already emerging, the explosion of AI-powered products and services is likely still a few years away. This phase could extend well into the 2030s and beyond.
It's important to note that these phases overlap, and the timeline is speculative. The pace of AI development could accelerate or face unexpected challenges.
Understanding these similarities, differences, and potential timelines can help businesses and policymakers better prepare for the AI-driven future. It suggests that while we can learn from past technological revolutions, AI's unique characteristics may require new development, regulation, and adoption approaches.
Challenges and Opportunities in the AI Cycle
The AI landscape is evolving at a breakneck pace, presenting a complex interplay of challenges and opportunities that are reshaping the technology sector and beyond. As we progress through the IPA cycle, we're witnessing a transformation that goes far beyond traditional tech disruption.
The mounting environmental cost is one of the most pressing challenges in the current AI boom. The carbon footprint of training large AI models is staggering, with some estimates suggesting that training a single large language model can emit as much CO2 as five cars over their lifetimes. This environmental impact is driving a new wave of innovation in green AI, with researchers and companies exploring novel ways to make AI more energy-efficient. From developing specialized AI chips that consume less power to creating algorithms that require less computational resources, the push for sustainable AI is opening up new frontiers in computer science and engineering.

Simultaneously, the AI talent crunch is reaching critical levels. The demand for AI specialists far outstrips supply, with some reports indicating that there are only about 300,000 AI researchers and practitioners worldwide to fill millions of roles. This scarcity is not only driving up salaries but also reshaping education and training paradigms. We're seeing the emergence of AI-focused coding bootcamps, online courses, and even AI-assisted programming tools designed to bridge this gap. This democratization of AI knowledge could lead to a more diverse and innovative AI landscape in the coming years.
The rapid advancement of AI is also outpacing our ability to govern it effectively. The regulatory landscape for AI is still in its infancy, with policymakers struggling to keep up with its ethical and societal implications. This regulatory lag is creating a unique opportunity for companies and researchers to shape the future of AI governance. We're seeing the rise of AI ethics boards within corporations, interdisciplinary research groups tackling AI policy, and novel approaches to algorithmic transparency and accountability.
The scale of AI models is growing at an astonishing rate, presenting both challenges and opportunities. For instance, GPT-3, released in 2020, was trained on 175 billion parameters - a number that seemed staggering at the time. Its successor, GPT-4, while specifications remain undisclosed, is believed to be significantly larger and more complex. This rapid scaling demonstrates the industry's push for more powerful models, but it also intensifies concerns about computational resources, energy consumption, and the environmental impact of AI training. At the same time, it opens up new possibilities for AI capabilities and previously thought impossible applications.
Perhaps one of the most intriguing developments is the potential for AI to solve its own limitations. As we push the boundaries of what's possible with current AI technologies, we're also using AI to improve AI itself. From neural architecture search algorithms that design better neural networks to AI systems that can explain their own decision-making processes, we're entering an era of recursive AI improvement. This self-improving cycle could lead to breakthroughs we can hardly imagine today.
The challenges in the AI cycle are not just technical but deeply human. As AI systems become more advanced, we're grappling with philosophical questions about the nature of intelligence, creativity, and even consciousness. This is spurring a renaissance in cognitive science and philosophy of mind, as researchers seek to understand human intelligence better to create more sophisticated AI.
In essence, the challenges and opportunities in the AI cycle are deeply intertwined, each problem presenting a new frontier for innovation. As we navigate this complex landscape, we're not just developing new technologies, but reshaping our understanding of intelligence, creativity, and what it means to be human in an AI-augmented world.
Future Outlook
As AI continues its journey through the IPA cycle, we stand on the cusp of a transformative era. While the exact path of AI development remains uncertain, we can discern some broad trends and potential developments that will shape our future.
The maturation of AI infrastructure will likely lead to more powerful, efficient, and accessible AI systems. As specialized AI hardware becomes more prevalent and cloud computing continues to evolve, we may see AI capabilities integrated into an ever-wider array of devices and services. This could lead to a world where AI assistance is as ubiquitous and unremarkable as electricity is today.
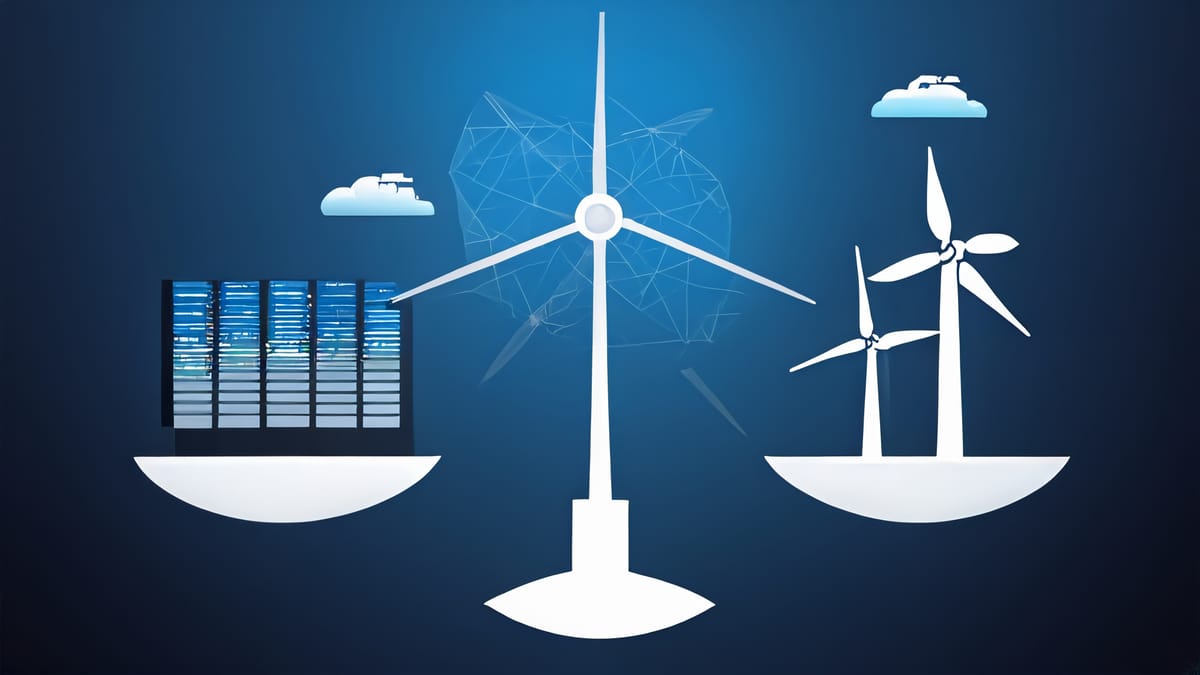
In the platform phase, we're likely to see AI development tools become increasingly sophisticated and user-friendly. This democratization of AI could lead to an explosion of innovation, with individuals and small teams able to create AI applications that today would require the resources of a large tech company. However, this also raises questions about the control and governance of AI technologies.
The application phase of AI holds perhaps the most exciting and unpredictable possibilities. Rather than trying to predict specific applications, it's more valuable to consider the broader implications of widespread AI adoption. We may see a fundamental shift in how we interact with technology, moving from explicit commands to more natural, contextual interactions. AI could become a collaborative partner in creative and problem-solving processes, augmenting human capabilities in ways we can barely imagine today.
However, this future also comes with significant challenges. As AI systems become more powerful and pervasive, issues of privacy, data ownership, and algorithmic bias will become increasingly critical. We'll need to grapple with complex ethical questions about the role of AI in decision-making processes that affect human lives.
The impact on the workforce is likely to be profound, but not necessarily in the way many predict. While AI will certainly automate many current jobs, history suggests that new technologies also create new types of work. The challenge will be in managing this transition, ensuring that the benefits of AI are broadly shared and that people have opportunities to adapt and upskill.
Perhaps most profoundly, the advancement of AI may lead us to reconsider fundamental questions about intelligence, consciousness, and what it means to be human. As AI systems become more sophisticated, the line between human and machine intelligence may become increasingly blurred, challenging our assumptions and potentially reshaping our societies fundamentally.
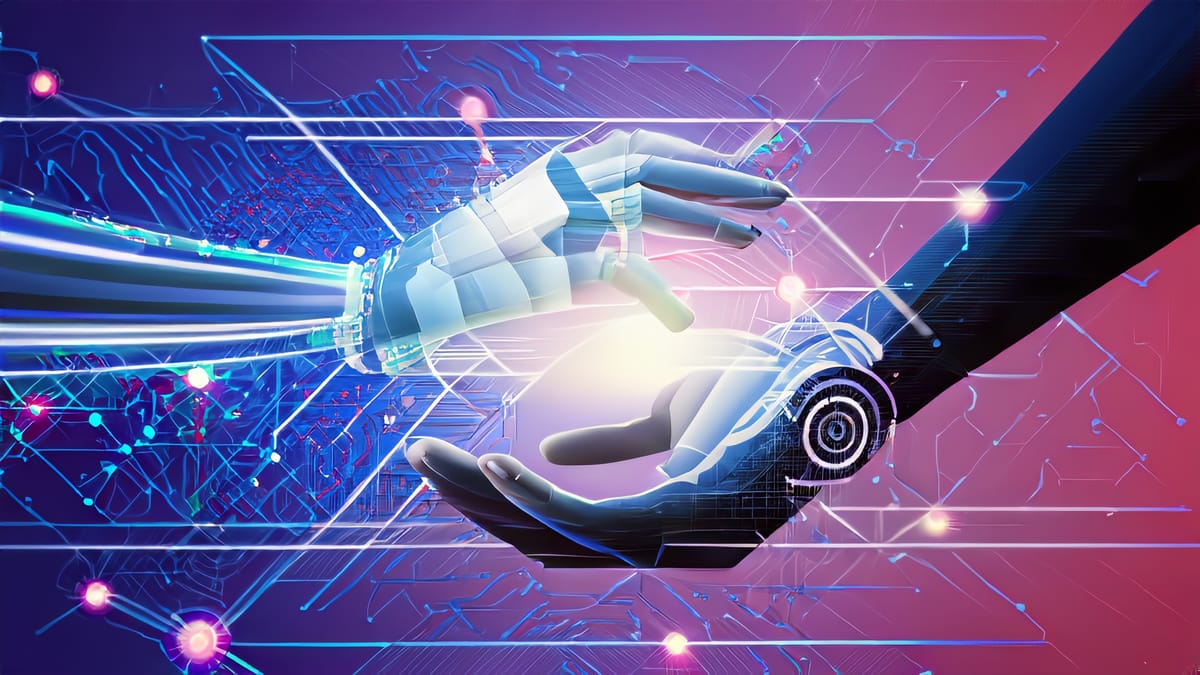
Flexibility and adaptability will be key to navigating this future. The most successful individuals, organizations, and societies will be those who can quickly learn and adjust to the rapidly changing landscape that AI creates. At the same time, we must not lose sight of our human values and ethical principles as we push the boundaries of what's technologically possible.
The future of AI is not predetermined. It's a future that we, collectively, have the power to shape. By understanding the IPA cycle and actively engaging with the development of AI technologies, we can work towards a future where AI enhances human capabilities, solves global challenges, and contributes to the betterment of society as a whole.
Conclusion
As we've explored throughout this analysis, the IPA (Infrastructure, Platforms, Applications) computing cycle provides a valuable framework for understanding the progression of AI technology. However, our examination reveals that AI's journey through this cycle is far from linear or predictable.
The current state of AI development is characterized by a unique acceleration and interconnectedness across all phases of the IPA cycle. We're witnessing simultaneous advancements in infrastructure, the rapid evolution of platforms, and the emergence of groundbreaking applications. This interconnected progress is creating a powerful feedback loop, driving innovation at an unprecedented pace.
The massive investments in AI infrastructure, from specialized hardware to expanded cloud capabilities, are laying the groundwork for increasingly sophisticated AI systems. Concurrently, the maturation of AI platforms is democratizing access to these powerful tools, enabling a broader range of developers and businesses to leverage AI capabilities. The rapid adoption of applications like ChatGPT demonstrates the immense potential and public appetite for AI-driven solutions, even as the underlying technologies continue to evolve.
This accelerated and interconnected development cycle presents both opportunities and challenges. For businesses and investors, it creates a landscape of rapid change where agility and foresight are crucial. The window for gaining a competitive advantage through AI adoption may be narrower than in previous technological shifts, emphasizing the importance of strategic and timely implementation.
Policymakers and regulators face the daunting task of creating frameworks that can keep pace with AI's rapid advancement while addressing critical issues such as data privacy, ethical use, and the societal impact of AI. The environmental implications of AI's growing computational demands also present a crucial challenge that requires innovative solutions.
Looking ahead, we can expect the boundaries between infrastructure, platforms, and applications in AI to blur further. We may see the emergence of integrated AI ecosystems that span all three phases, offering comprehensive solutions for AI development and deployment. This convergence could usher in a new paradigm in how we conceptualize and interact with AI technologies.
In conclusion, while we're still in the early stages of AI's transformative journey, the accelerated and interconnected nature of the current IPA cycle suggests that the most revolutionary changes may arrive sooner and more dramatically than many anticipate. As we move forward, it will be crucial for all stakeholders – from developers and businesses to policymakers and the general public – to remain engaged and adaptable in shaping the future of AI.
The AI landscape of the coming years promises to be one of rapid innovation, unexpected breakthroughs, and profound societal impact. By understanding and anticipating the dynamics of the IPA cycle in AI, we can better prepare for and harness the transformative potential of this revolutionary technology.
Thanks for reading,
Giro.